Navigating Common Data Science Pitfalls Of Customer Retention
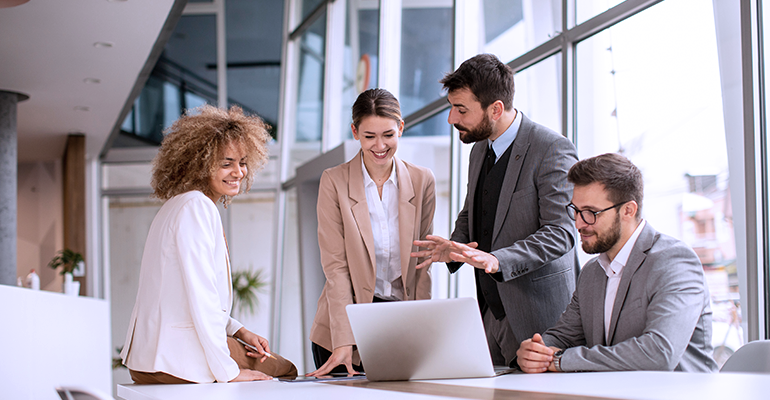
Navigating Common Data Science Pitfalls Of Customer Retention
In today’s customer-centric market, addressing customer churn is no less than a battle. It requires in-depth data-led customer insights for proactive identification of churn risks, driving timely preventive measures to retain valuable customers. And in this battle, data science is an indispensable weapon.
However, if not approached strategically, data-science-led retention exercises can result in undesired outcomes, wasted resources and unnecessarily high costs. In this blog, let us look at the factors that can make data science ineffective and uneconomical and what measures you can take to address those factors.
Poor Data Quality
If the data used for analysis is inaccurate, incomplete or outdated, the insights and predictions generated by data science models can be unreliable, rendering the obtained intelligence ineffective for customer retention strategies.
Solution: Prioritizing high-value data
Focus on collecting and analyzing data that directly impacts customer behavior and preferences, such as call center notes, transaction and service history, customer feedback, etc. It is important to invest in data quality early in the retention process to reduce errors downstream. This saves time and effort during analysis and leads to more effective retention insights.
Using A Single-Model Approach
This is a common pitfall committed by recurring revenue businesses while approaching customer churn. They often rely on a single-model strategy that focuses on the end of the term of customers. The problem with this approach is that it is often too late to address churn risk by then. Besides, a single machine learning model can answer only one question at a time and fails to look at the complete customer journey. Therefore, this classification alone is insufficient to reduce churn.
Solution: Use multiple models for a lifecycle-based approach
Each customer’s journey is unique and multiple factors over the customer lifecycle drive the desire to cancel. That is why you should use multiple predictive models. These models should focus on every stage of the entire lifecycle to uncover risks and opportunities throughout, helping predict a complete and accurate dimension of customer behavior. This means more actionable intelligence and better ROI on your data science investment.
Lack Of Collaboration.
Effective data science for customer retention necessitates a seamless integration of cross-functional teams, including data analysts, domain experts, marketers and customer support to share insights and align strategies. When these teams work in isolation or fail to collaborate effectively, valuable insights may remain untapped, leading to inefficient resource allocation and missed opportunities for customer retention. In turn, this lack of collaboration leads to increased costs, diminishing the economic viability of data science initiatives.
Solution: Bring all skills under one umbrella.
You need to establish a collaborative approach to address this problem. Ensure that all relevant departments, i.e., data scientists, data engineers, domain experts and stakeholders collaborate closely so that data-driven insights are relevant and actionable and align with business realities.
Ineffective Communication
Effective communication is a cornerstone of successful data science. Even the most accurate and insightful data science models are of little use if the findings cannot be effectively communicated to decision-makers. If the alignment between the data science team and customer retention objectives is missing, it can result in wasted time and resources as well as missed opportunities for leveraging data to drive improvements.
Solution: Communicate the right insights to decision-makers
Fostering a culture of effective communication within organizations is essential to maximize the economic benefits of data science. Ensure clear and concise communication of results to decision-makers to turn data into actionable strategies, make effective business decisions and realize the full potential of your customer data.
Lack Of Required Skills
Implementing an effective data-powered retention solution requires a team of experts with multidisciplinary skill sets, including statistics, programming, domain knowledge and data manipulation. These experts are often in high demand and can be costly to hire and retain. Additionally, the data infrastructure required for robust data science operations can be expensive to set up and maintain.
Solution: Partnering with an expert
For many organizations, comprehensive capabilities, including necessary talent and infrastructure, are unavailable or uneconomical in-house. In that case, partnering with a data-science-powered retention firm can be a prudent choice. It not only helps you harness the power of data science but also provides you with external expertise, cutting-edge tools, methodologies and insights tailored to your specific needs
The Need For Continuous Improvement
Customer behaviors and preferences evolve over time and the pace of this change is unprecedentedly fast today. Customers are more demanding and the competition is intense. This is a major challenge for subscription businesses since many of them fail to adapt their data science models to these continuous changes, which leaves the overall retention strategy less effective than desired.
Solution: Implement a process for continuous monitoring
To tackle this challenge, you should be on top of the changes in customer behavior and expectations. Monitor the performance of your predictive models and update them based on new data and changing trends. Consider using A/B testing and experimentation to validate the effectiveness of different retention approaches.
An economical and effective data science approach involves optimizing the use of resources while still achieving valuable insights and outcomes. It involves striking a balance between your analytical capabilities and financial prudence. Once that happens, you can harness the benefits of data-driven customer retention without incurring unnecessary expenses. By following the principles listed above, you can achieve meaningful insights and outcomes without overspending on unnecessary tools or processes.